GPT-4.1 and o4-mini: Is OpenAI Overselling Long-Context?
We put OpenAI’s latest models through the LongMemEval benchmark—here’s why raw context size alone isn't enough.
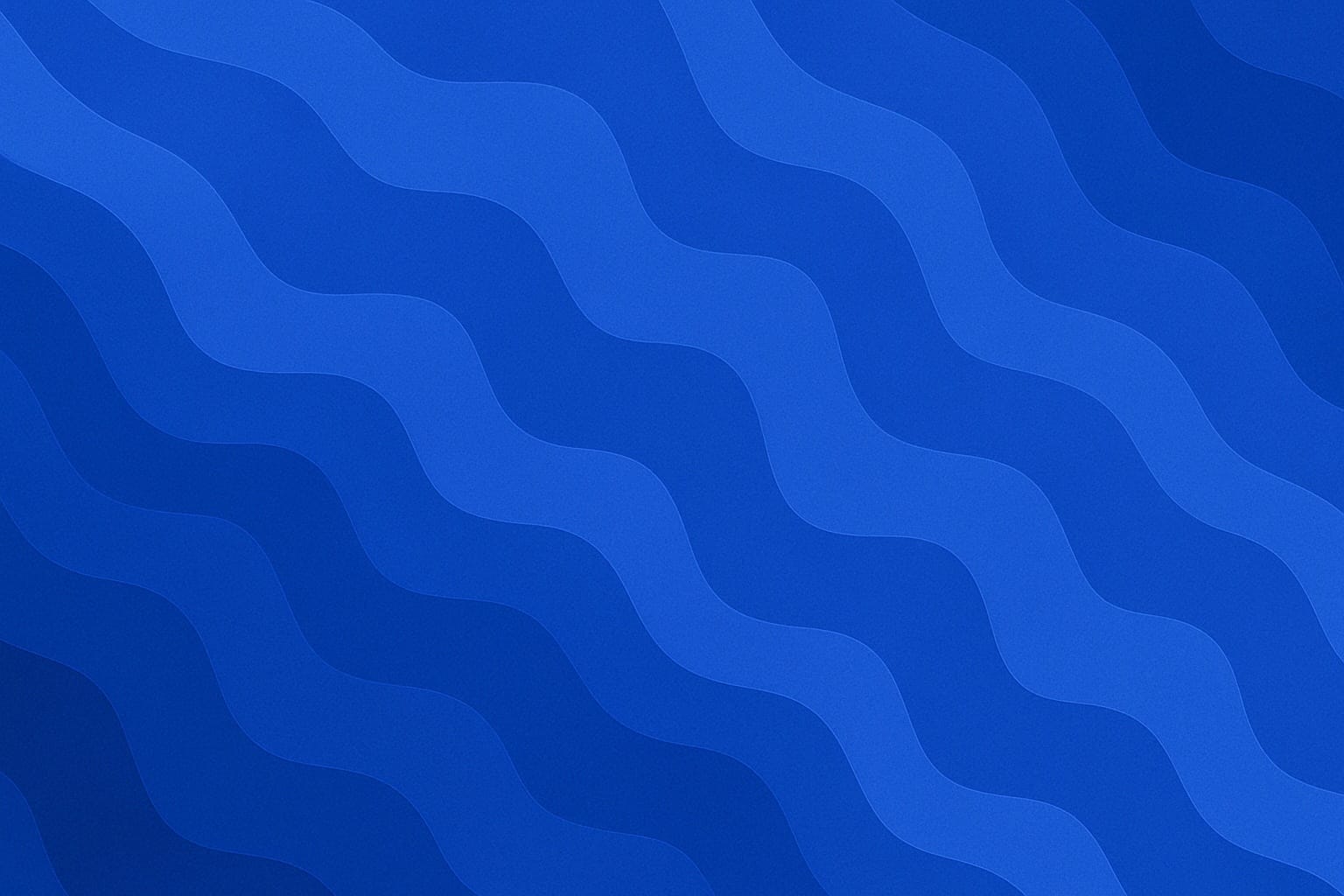
OpenAI has just released several new models: GPT-4.1, the company's new flagship model, GPT-4.1 mini, and GPT-4.1 nano, alongside the reasoning-focused o3 and o4-mini models. These releases came with impressive claims around improved performance in instruction following and long-context capabilities. Both GPT-4.1 and o4-mini feature expanded context windows, with GPT-4.1 supporting up to 1 million tokens of context.
We recently published a paper introducing Zep, a knowledge graph-based memory system for AI agents. In that study, Zep showed clear performance gains on the LongMemEval benchmark compared to the common practice of placing all evaluation data directly into the context window. In this follow-up, we run OpenAI's latest models through the same LongMemEval tests to see how their enhanced context handling stacks up against our knowledge graph approach.
The LongMemEval Benchmark
LongMemEval, introduced at ICLR 2025, is a comprehensive benchmark designed to evaluate the long-term memory capabilities of chat assistants across five core abilities:
- Information Extraction: Recalling specific information from extensive interactive histories
- Multi-Session Reasoning: Synthesizing information across multiple history sessions
- Knowledge Updates: Recognizing changes in user information over time
- Temporal Reasoning: Awareness of temporal aspects of user information
- Abstention: Identifying when information is unknown
We specifically used the LongMemEval_S
dataset for this evaluation. Each conversation in this dataset averages around 115,000 tokens—about 10% of GPT-4.1’s maximum context size of 1 million tokens and roughly half the capacity of o4-mini. We decided against benchmarking the o3 model because its evaluation cost would have been prohibitively high.
The benchmark includes six question types that map to these abilities discussed above:
- Single-session-user:
Tests if the model remembers specific details provided by the user during a recent conversation. It’s the simplest form of memory, focused on immediate recall. - Single-session-assistant:
Checks if the model recalls its own previous statements or advice from within the same session, essential for conversation consistency. - Single-session-preference:
Evaluates how well the model recognizes user preferences mentioned earlier in a conversation and applies them to personalize recommendations or responses. - Multi-session:
Measures the model's ability to combine and use information mentioned across multiple distinct conversation sessions, important for maintaining long-term context. - Knowledge-update:
Tests if the model correctly detects and adapts to updates in user information over time—like changes in location or employment. - Temporal-reasoning:
Assesses whether the model accurately interprets time-related references (e.g., "last month," "two weeks ago") and timestamps, and understands the sequence of events.
Together, these evaluations comprehensively measure a model’s ability to maintain accurate, useful long-term memory—key to creating personalized and coherent AI assistants.
Methodology
We evaluated three new configurations on LongMemEval:
- GPT-4.1 with full context
- GPT-4.1 with a modified prompt
- O4-mini with full context
We compared these against previously reported results for GPT-4o, GPT-4o-mini, and Zep (powered by GPT-4o). To maintain consistency, GPT-4o served as the judge model across all new evaluations, matching our earlier methodology.
Initial tests revealed that GPT-4.1 struggled with the standard evaluation prompt, so we adjusted the instructions to improve its performance. The original prompt was:
Your task is to briefly answer the question.
You are given the following context from the previous conversation.
If you don't know how to answer the question, abstain from answering.
The modified prompt adding an explicit instruction to infer answers from the context:
You should use the information from the context to infer the answers to questions.
For example when asked questions about how often, how many, or how many times,
you should assume all necessary information is in the context.
This modification aligns with OpenAI's GPT-4.1 prompting guide, which notes that "GPT-4.1 is trained to follow instructions more closely and more literally than its predecessors."
Results
Here are the accuracy results across all evaluated models:
Question Type | gpt-4o-mini | gpt-4o | gpt-4.1 | gpt-4.1 (mod) | o4-mini | Zep gpt-4o |
---|---|---|---|---|---|---|
single-session-preference | 30.0% | 20.0% | 16.67% | 16.67% | 43.33% | 56.7% |
single-session-assistant | 81.8% | 94.6% | 96.43% | 98.21% | 100.00% | 80.4% |
temporal-reasoning | 36.5% | 45.1% | 51.88% | 51.88% | 72.18% | 62.4% |
multi-session | 40.6% | 44.3% | 39.10% | 43.61% | 57.14% | 57.9% |
knowledge-update | 76.9% | 78.2% | 70.51% | 70.51% | 76.92% | 83.3% |
single-session-user | 81.4% | 81.4% | 65.71% | 70.00% | 87.14% | 92.9% |
Average | 57.87% | 60.60% | 56.72% | 58.48% | 72.78% | 72.27% |
Analysis
o4-mini: Strong Reasoning Makes the Difference
o4-mini clearly stands out in our evaluation, achieving the highest overall average score of 72.78%. Its strong performance supports OpenAI’s claim that the model is optimized to "think longer before responding," making it especially good at tasks involving deep reasoning.
In particular, o4-mini shines in temporal reasoning tasks (72.18%) and hits perfect accuracy on single-session assistant questions (100%). These results highlight its strength at analyzing context and reasoning through complex memory-based problems.
Zep’s Knowledge Graph: Closing the Gap with o4-mini
Zep, using GPT-4o, achieved an average accuracy of 72.27%, essentially matching o4-mini—even though it relied on the older model for both graph-building and knowledge retrieval. This underscores how Zep’s knowledge graph architecture, which precomputes knowledge extraction and temporal information, effectively compensates for limitations in the base model’s capabilities.
Zep particularly stands out in these areas:
- Single-session preference (56.7%): Clearly outperforming approaches that rely purely on raw context.
- Knowledge update (83.3%): Leading across the board when it comes to handling updates to user information over time.
- Single-session user (92.9%): Best performance in straightforward information extraction tasks.
One category where Zep trails is single-session assistant tasks (80.4%). Zep’s inability to handle very large assistant messages in this test resulted in message truncation, and information being lost. We plan to improve this by implementing message splitting in future updates.
GPT-4.1: Bigger Context Isn’t Always Better
Despite its large 1M-token context window, GPT-4.1 underperformed with an average accuracy of just 56.72%—lower even than GPT-4o-mini (57.87%). Modifying the evaluation prompt improved results slightly (58.48%), but GPT-4.1 still trailed significantly behind o4-mini and Zep.
These results suggest that context window size alone isn’t enough for tasks resembling real-world scenarios. GPT-4.1 excelled at simpler single-session-assistant tasks (96.43%), where recent context is sufficient, but struggled with tasks requiring simultaneous analysis and recall. At this stage, we're unsure whether poor performance was as a result of improved instruction adherence or the potentially deleterious effects of increasing the context window size.
GPT-4o: Solid But Unspectacular
GPT-4o achieved an average accuracy of 60.60%, making it the third-best performer. While it excelled at single-session-assistant tasks (94.6%), it notably underperformed on single-session-preference (20.0%) compared to both o4-mini (43.33%) and Zep (56.7%).
Practical Implications
These results have several implications for engineering teams building applications with these models:
- Specialized reasoning models matter: o4-mini demonstrates that even small models specifically trained for reasoning tasks can significantly outperform general-purpose models with larger context windows in recall-intensive applications.
- Knowledge graphs remain valuable: Despite advances in model capabilities, Zep's knowledge graph approach, using gpt-4o, delivers nearly identical performance to o4-mini while using the much older, general-purpose model. This suggests structured knowledge representations continue to offer significant advantages.
- Raw context size isn't everything: GPT-4.1’s disappointing performance despite its 1M-token context highlights that simply expanding the context size doesn't automatically improve large-context task outcomes. Additionally, GPT-4.1’s stricter adherence to instructions may sometimes negatively impact performance compared to earlier models such as GPT-4o.
Latency and Cost Considerations
Accuracy isn’t the only factor to consider. Our previous evaluation showed that Zep reduces response latency by about 90% compared to to filling the context window. This remains a significant advantage, even against the newer models, since processing the benchmark's full 115,000-token context introduces substantial latency and cost. In contrast, Zep achieves comparable accuracy by retrieving only around 2% of that context.
For production applications, Zep’s combination of fast responses, low context usage, and competitive accuracy makes it particularly appealing when both cost efficiency and quick response times matter.
Conclusion
Our evaluation highlights that o4-mini and Zep currently offer the best approaches for applications that rely heavily on recall, including memory use cases. While both achieved similar overall results, each excelled differently across specific tasks.
Interestingly, GPT-4.1’s larger context window didn't translate into better recall performance, demonstrating that how effectively models reason over and utilize context is more important than raw context size. This insight should inform how teams select models for real-world tasks requiring strong recall capabilities.
For engineering teams, these findings suggest two practical pathways:
- o4-mini is well-suited to applications emphasizing single-session assistant recall and temporal reasoning.
- Zep’s knowledge graph architecture excels in scenarios needing user preference understanding, accurate knowledge updates, reliable information extraction, and particularly where low latency and cost-efficiency matter. Zep achieves these benefits while retrieving just 2% of the context used by full-context approaches.
We’re actively working to integrate GPT-4.1 and o4-mini into Zep and anticipate substantial improvements in recall performance from combining structured knowledge representations with advanced reasoning models.
Resources
- Zep: A Temporal Knowledge Graph Architecture for Agent Memory: Our research paper detailing the architecture and evaluation. arXiv:2501.13956
- Graphiti: The temporal knowledge graph engine powering Zep. Graphiti GitHub repository
- LongMemEval: Benchmarking Chat Assistants on Long-Term Interactive Memory: Comprehensive benchmark for evaluating long-term memory capabilities of LLM-based assistants. arXiv:2410.10813
- GPT-4.1 Model Family: Technical details and capabilities of OpenAI's newest model series. OpenAI Blog
- GPT-4.1 Prompting Guide: Official guide to effectively prompting GPT-4.1. OpenAI Cookbook
- O3 and O4-mini: Announcement and technical details of OpenAI's reasoning-focused models. OpenAI Blog