Lies, Damn Lies, & Statistics: Is Mem0 Really SOTA in Agent Memory?
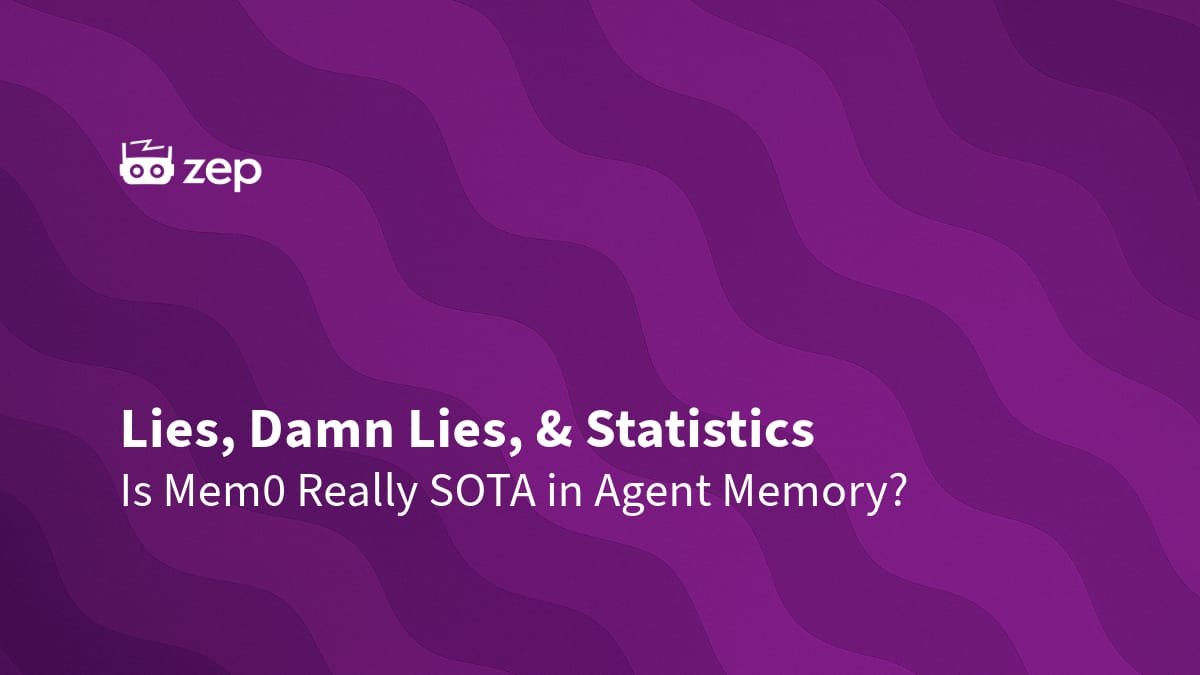
Mem0 recently published research claiming to be the State-of-the-Art in Agent Memory, besting Zep. In reality, Zep outperforms Mem0 by 10% on their chosen benchmark. Why the discrepancy? We dig in to understand.
Recently, Mem0 published a paper benchmarking their product against competitive agent memory technologies, claiming state-of-the-art (SOTA) performance based on the LoCoMo benchmark.
Benchmarking products is hard. Experimental design is challenging, requiring careful selection of evaluations that are adequately challenging and high-quality—meaning they don't contain significant errors or flaws. Benchmarking competitor products is even more fraught. Even with the best intentions, complex systems often require a deep understanding of implementation best practices to achieve best performance, a significant hurdle for time-constrained research teams.
Closer examination of Mem0’s results reveal significant issues with the chosen benchmark, the experimental setup used to evaluate competitors like Zep, and ultimately, the conclusions drawn.
This article will delve into the flaws of the LoCoMo benchmark, highlight critical errors in Mem0's evaluation of Zep, and present a more accurate picture of comparative performance based on corrected evaluations.
Zep Significantly Outperforms Mem0 on LoCoMo (When Correctly Implemented)
When the LoCoMo experiment is run using a correct Zep implementation (details below and see code), the results paint a drastically different picture.
Our evaluation shows Zep achieving an 75.14% +/- 0.17 J score, significantly outperforming Mem0's best configuration (Mem0 Graph) by approximately 10% relative improvement. This starkly contrasts with the 65.99% score reported for Zep in the Mem0 paper, likely a direct consequence of the implementation errors discussed below.
Search Latency Comparison (p95 Search Latency):
Focusing on search latency (the time to retrieve relevant memories), Zep, when configured correctly for concurrent searches, achieves a p95 search latency of 0.632 seconds. This is faster than the 0.778 seconds reported by Mem0 for Zep (likely inflated due to their sequential search implementation) and slightly faster than Mem0's graph search latency (0.657s).
While Mem0's base configuration shows a lower search latency (0.200s), it's important to note this isn't an apples-to-apples comparison; the base Mem0 uses a simpler vector store / cache without the relational capabilities of a graph, and it also achieved the lowest accuracy score of the Mem0 variants.
Zep's efficient concurrent search demonstrates strong performance, crucial for responsive, production-ready agents that require more sophisticated memory structures. Note: Zep's latency was measured from AWS us-west-2 with transit through a NAT setup.
Why LoCoMo is a Flawed Evaluation
Mem0's choice of the LoCoMo benchmark for their study is problematic due to several fundamental flaws in the evaluation's design and execution:
Tellingly, Mem0's own results show their system being outperformed by a simple full-context baseline (feeding the entire conversation to the LLM)..
- Insufficient Length and Complexity: The conversations in LoCoMo average around 16,000-26,000 tokens. While seemingly long, this is easily within the context window capabilities of modern LLMs. This lack of length fails to truly test long-term memory retrieval under pressure. Tellingly, Mem0's own results show their system being outperformed by a simple full-context baseline (feeding the entire conversation to the LLM), which achieved a J score of ~73%, compared to Mem0's best score of ~68%. If simply providing all the text yields better results than the specialized memory system, the benchmark isn't adequately stressing memory capabilities representative of real-world agent interactions.
- Doesn't Test Key Memory Functions: The benchmark lacks questions designed to test knowledge updates—a critical function for agent memory where information changes over time (e.g., a user changing jobs).
- Data Quality Issues: The dataset suffers from numerous quality problems:
- Unusable Category: Category 5 was unusable due to missing ground truth answers, forcing both Mem0 and Zep to exclude it from their evaluations.
- Multimodal Errors: Questions are sometimes asked about images where the necessary information isn't present in the image descriptions generated by the BLIP model used in the dataset creation.
- Incorrect Speaker Attribution: Some questions incorrectly attribute actions or statements to the wrong speaker.
- Underspecified Questions: Certain questions are ambiguous and have multiple potentially correct answers (e.g., asking when someone went camping when they camped in both July and August).
Given these errors and inconsistencies, the reliability of LoCoMo as a definitive measure of agent memory performance is questionable. Unfortunately, LoCoMo isn't alone; other benchmarks such as HotPotQA also suffer from issues like using data LLMs were trained on (Wikipedia), overly simplistic questions, and factual errors, making robust benchmarking a persistent challenge in the field.
Mem0's Flawed Evaluation of Zep
Beyond the issues with LoCoMo itself, Mem0's paper includes a comparison with Zep that appears to be based on a flawed implementation, leading to an inaccurate representation of Zep's capabilities:
- Incorrect User Model: Mem0 utilized a user graph structure designed for single user-assistant interactions but assigned the user role to both participants. This likely confused Zep's internal logic, treating it as a single user whose identity changed with each message.
- Improper Timestamp Handling: Timestamps were passed by appending them to messages, rather than using Zep's dedicated created_at field. This non-standard method would interfere with Zep's temporal reasoning capabilities.
- Sequential vs. Parallel Searches: Searches were performed sequentially instead of in parallel, artificially inflating Zep's reported search latency in Mem0's results.
These implementation errors fundamentally misrepresent how Zep is designed to function, inevitably leading to the suboptimal performance reported in Mem0's paper.
The Need for Better Benchmarks: Why Zep Prefers LongMemEval
The issues with LoCoMo underscore the need for more robust and realistic benchmarks. The Zep team prefers evaluations like LongMemEval, which addresses many of LoCoMo's shortcomings:
- Length and Challenge: Features significantly longer conversations (avg. 115k tokens), truly pushing context limits.
- Temporal Reasoning & State Changes: Explicitly tests temporal understanding and the ability to handle changing information (knowledge updates).
- Quality: Human-curated and designed to be high quality.
- Enterprise Relevance: Better represents the complexity and demands of real-world enterprise use cases.
Zep has demonstrated strong performance on LongMemEval, achieving significant accuracy improvements and latency reductions compared to baselines, particularly on complex tasks like multi-session synthesis and temporal reasoning.
Conclusion
Benchmarking is challenging, and evaluating competitor products requires diligence and expertise to ensure fair and accurate comparisons. The Mem0 paper's claims of SOTA performance appear to be based on a flawed benchmark (LoCoMo) and a demonstrably incorrect implementation of a competitor system (Zep).
When evaluated correctly on the same benchmark, Zep significantly outperforms Mem0 in accuracy and demonstrates highly competitive search latency, especially when comparing graph-based implementations. This discrepancy highlights the critical importance of rigorous experimental design and understanding the systems under evaluation.
Moving forward, the field needs better, more representative benchmarks. We encourage the Mem0 team to evaluate their product on more challenging and realistic benchmarks like LongMemEval, where Zep has published its results, to facilitate a more meaningful comparison of long-term memory capabilities for AI agents.
Next Steps
- Read the paper Zep: A Temporal Knowledge Graph Architecture for Agent Memory
- View the code for this analysis: GitHub repo
- Sign up for a Zep account